At IBC 2023, in addition to presenting its private 5G and HDR conversion solutions, b<>com will be unveiling *Wisdom*, an AI-driven energy-saving broadcast solution that allows intelligent frame rate optimization. Learn all about b<>com’s latest innovative broadcast technology from Tania Pouli, researcher and deputy director of the Image, Vision & Immersion Laboratory at b<>com.
At IBC, you'll be unveiling b<>com *Wisdom*. Can you tell us what it's all about?
b<>com *Wisdom* is a solution that adapts frame rate to content.
In concrete terms, thanks to a technology based on Artificial Intelligence (AI), we propose to remove images from videos to save storage and energy, but without losing the quality of the original content. We only remove images whose deletion is imperceptible to the viewer.
How did you get the idea to work on this algorithm?
When the new UHD formats were defined, we were asked to produce content for our own research work in the Image Vision & Immersion lab.
We worked on motion blur, and filmed simultaneously with two cameras: one at 60 fps (60 frames per second, the reference value) and the other at 120 fps (high frame rate for greater fluidity in games, for example).
This enabled us to compare the resulting videos, and to highlight the benefits of high frame rates for certain types of content. But it also showed that for certain scenes, increasing the frame rate was of no help at all, hence the idea of varying it dynamically according to the content!
When you describe your solution as "intelligent", you mean that it works with AI. What role does it play?
Our solution is based on an artificial intelligence model that enables us to quickly select the ideal frame rate for each group of four images. Working in groups of 4 images enables us to be highly responsive to changes in content. AI gives us the ability to know precisely when it's possible to delete images without it being noticeable, thanks to a model that has been learned from thousands of images and takes into account multiple characteristics extracted from the content.
Who is this innovation aimed at? And what are the concrete benefits for future users?
This technology is aimed at both content creators (studios and TV channels) and distribution platforms (Orange/Netflix/Disney/etc.).
Our solution can be used at different points in the content creation process:
It can be used at the post-production stage, to avoid manipulating excessive amounts of data. The solution can be seen as a zip-unzip when storing videos in mezzanine format, with file size reductions of up to 40%.
Another application is content distribution. Here, we're dealing with broadcast formats such as MPEG-AVC or HEVC, and the reduction in bitrate is 5-15%. This reduction is more moderate, however, since the images removed are not intended to be used as references, and are therefore B images of reduced size.
How is this solution different from what already exists on the market?
The main advantage of our solution is that it varies the frame rate according to the content, whereas other technologies do so according to other criteria such as bandwidth or computing power.
As a result, our solution stands out for its ability to preserve the quality of experience, since processing is imperceptible.
What's more, our technology complements rather than competes with other approaches.
For example, if we take the adaptive bitrate (ABR) commonly used for video streaming, each video segment, which is encoded according to different formats, can very well then be processed with our technology so as to improve the overall video quality distributed to subscribers for a given distribution capacity.
How is this solution different from what already exists on the market?
The main advantage of our solution is that it varies the frame rate according to the content, whereas other technologies do so according to other criteria such as bandwidth or computing power.
As a result, our solution stands out for its ability to preserve the quality of experience, since processing is imperceptible.
What's more, our technology complements rather than competes with other approaches.
For example, if we take the adaptive bitrate (ABR) commonly used for video streaming, each video segment, which is encoded according to different formats, can very well then be processed with our technology so as to improve the overall video quality distributed to subscribers for a given distribution capacity.
Let's say I'm the representative of a content distributor. What would you say to convince them to use your solution?
I'd explain that, although some video encoders already offer quite similar treatments and drastically reduce the bitrate of certain frames (particularly B frames), we offer them the possibility of choosing which frames they can afford to remove. And that makes all the difference to the final rendering of the content! Our solution is the only one that both respects the initial quality of the content and optimizes image savings. This was confirmed by our subjective test campaign, which confirmed that the processing carried out does not change the video quality perceived by subscribers.
Ultimately, it will save storage capacity on its servers, with no loss of content quality.
You emphasize the frugality of your solution. Can you elaborate on this point? Do you have any figures to share with us?
When we apply b<>com *Wisdom* before compression, the gain on the size of files to be stored or exchanged is around 35%.
If we apply it to already encoded videos, the gain in throughput is more moderate, from 5% to 15% depending on the case, but there's also an impact on the receiver side that's difficult to quantify because it depends so much on the type of hardware implementation.
In any case, it's instinctive to think that removing images that contribute nothing can only be a good thing!
The same technology can be used on the source before encoding, saving up to 40% of uncompressed images to store and/or distribute to content providers.
What are the next developments planned?
We'd like to integrate the solution into a CDN so that we can measure the benefits in real-life conditions.
If we can show how many more users we can serve with the same infrastructure, then we'll be able to quantify the benefits of the solution even more precisely.
At the same time, we're continuing to think about ways of developing the algorithm that could have an impact on other video parameters...
More info on the Image Vision & Immersion lab
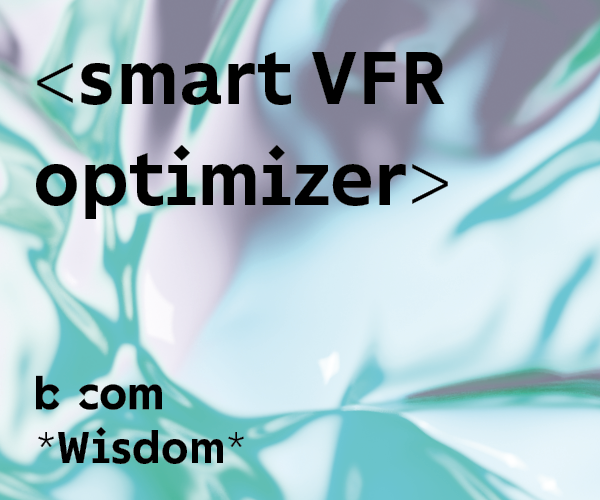