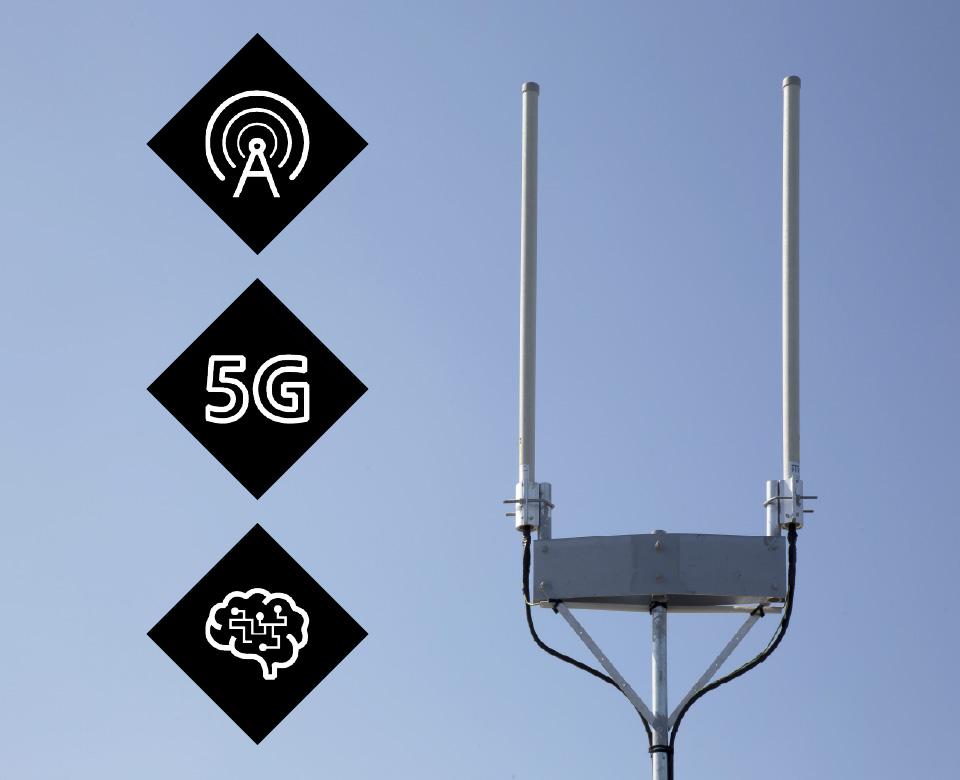
The challenge of decarbonizing 5G mobile networks for network operators
Optimizing power consumption for greater 5G energy efficiency
Reducing the energy consumption of 5G systems depends on overcoming several technological hurdles:
Unified data management:
The diversity of data sources generates a mass of heterogeneous information that is difficult to exploit. The following types of data are particularly concerned :
- Radio Intelligent Controller (RIC) at gNodeB level,
- OAM (Operations, Administration, and Maintenance) data,
- Network probes (IP flow measurements, loss rates, QoS)
- NwDAF (Network Data Analytics Function) connected to core functions (AMF, SMF, UPF, PCF, UDM, etc.).
Environment modeling:
Creating a digital twin of 5G networks is emerging as a key solution to maximize spectral efficiency and reduce the energy footprint of mobile networks. This digital twin makes it possible to simulate and optimize advanced energy-focusing technologies essential for more sustainable connectivity. However, this innovative approach still poses significant technical challenges, particularly regarding numerical control and real-time estimation, two crucial steps that remain greedy in computing resources. Overcoming these obstacles is essential to exploiting this technology's full potential and accelerating the transition to more responsible telecom networks.
Optimizing cloud orchestration:
In virtualized infrastructures, orchestrators must reconcile quality of service (QoS) and energy efficiency through auto-scaling policies (also called automatic scaling, a cloud computing feature that automatically allocates computing resources according to system demand). These mechanisms are based on detailed modeling of complex interactions.
Design compromises to guarantee frugal, high-performance AI
Frugal AI optimizes the amount of data exploited, facilitates the construction of algorithms, guides the choice of hardware and the energy source used in order to achieve a reduction in the environmental impact of AI, while at the same time maintaining its performance.
Technically, it reduces the complexity of algorithms in real-time functions, and therefore the amount of energy expended in these algorithms. Real-time signal processing relies on a delicate compromise between statistical optimization and algorithm simplicity. This is a subject that is difficult to reconcile with equipment limited in memory and computing power.
At the same time, neural networks have come into their own in recent years. They can be used for a wide range of tasks, such as classification, prediction or automatic translation, but they do not always meet certain statistical requirements, such as bias reduction. The design of these networks can be based on simulated data. This opens the way to a technological breakthrough in eco-design, by making it possible to develop explainable embedded AI, specifically adapted to the constraints of 5G systems.
Predictive maintenance on long-distance optical networks aims to analyze alarms generated by equipment, while exploiting latency and power measurements to anticipate failures. This helps prevent service degradation, limit costly interventions and improve infrastructure reliability. However, exploiting the massive databases generated by optical networks is one of the main challenges for predictive maintenance. This is due to the sheer volume of data to be processed, the lack of contextualization of relationships between data, and a lack of clear documentation, which hinders data interpretation.
The advantage of the innovation circle, at the crossroads of industrial needs and business expertise
The project led by b<>com, Mitsubishi Electric, Orange, TDF and INSA is distinguished by an approach in which each member brings specific expertise to a key link in the value chain. From the identification of use cases to business expertise and access to data, this complementarity enables us to cover all the issues linked to the decarbonization of mobile networks, while maximizing their efficiency and impact.
Mitsubishi Electric and Orange have operational use cases in a wide range of mobile network professions:
- Energy production system and life cycle analysis for Mitsubishi ;
- Network operator for Orange.
They each benefit from several years' worth of archived data.
b<>com has a high-performance computing center, with an industrial transfer mission, and brings scientific skills in AI, digital information processing, applied mathematics and statistics.
Key benefits for network operators
The platform developed within the b<>com project will make it possible to:
- Reduce RAN energy consumption by 50% compared with today, thanks to eco-design and virtualization;
- Better allocate tasks in the 5G core and in the cloud to save consumption, while maintaining good quality of service;
- Develop predictive maintenance for long-distance networks to reduce maintenance costs by 30%;
- Analyze the life cycles of certain components, and propose reprocessing chains;
- Increase equipment lifespan by 100% through eco-design.
Does this project give you ideas? To find out more about how you can work with b<>com, or to join our innovation circle, please contact our experts.