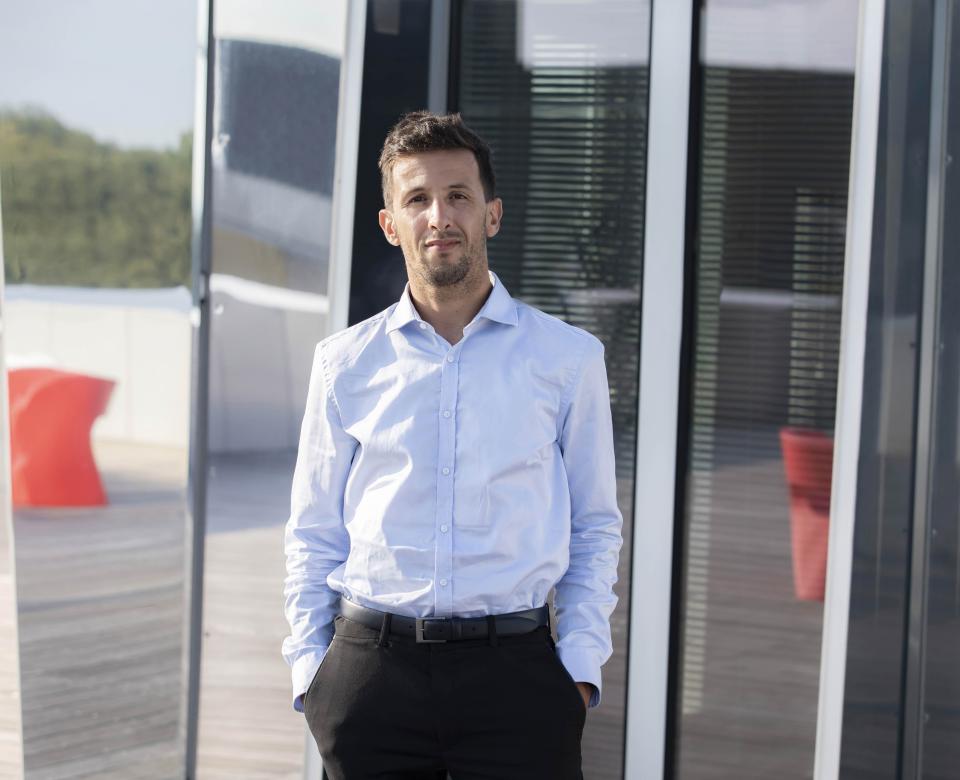
For several years, digital content has grown steadily, particularly on social media and digital sharing platforms. In 2020, YouTube saw about 500 hours of video published each minute. On Instagram, users post 100 million photos and videos on average per day!
As digital technology becomes a bigger part of our modern societies each day, the authenticity of data has come to be essential to preserving social order. Unfortunately, the emergence of new technologies like deepfakes has drastically altered that authenticity, and threatens our trust in digital content. In 2021, it is estimated that more than one out of every two videos will be bogus.
What is a “deepfake”?
Rigging, photoshopping, manipulating, or retouching images and videos has been a common practice for decades: The first version of Photoshop 4.0 came out in 1996. Although that software could generate synthetic multimedia content with heavy manual user involvement, new techniques for doing so are both fully automated and extremely realistic-rendering. This results in a sort of hyper-manipulation. The deepfake is one of these new technologies.
The word deepfake comes from “deep” as in deep learning, and "fake” to indicate falsified content, which leads to the following definition: “Deep learning-based creation of fake content”.
Originally, this term appeared in autumn 2017 with reference to a hyperrealistic face swapping technology.
The basic principle is learning the facial characteristics of two people, a source and a target, with an autoencoding paradigm. The face replacement is achieved by cascading the source decoder with the target encoder. In other words, the dynamics of the source face movements are retargeted onto the target.
Since then, other approaches have emerged, such as generative adversarial nets that have been developed into four main families: Full face synthesis, face attributes editing, face reenactment, and face swapping. The same strategies can also be transposed into another modality such as audio, by synthesizing or changing the expressiveness of a voice.
What impacts could deepfakes have on society?
In 2019, users spent an average of 144 minutes a day on social media, up 60% from 2012. The influence of digital content on individual and collective behavior has become inescapable. With the added ingredient of deepfakes, trust in digital media is under threat.
Let’s take politics as an example. If deepfakes were to become common there, the influence of public opinion would become larger and harder to track, thereby leading to biased strategic decisions.
The obiquitous of deepfakes could create a feeling of powerless when it comes to figuring out what content is real, leading to a loss of trust in digital information. If this sentiment were to gradually take root, it would throw a chaotic element into traditional collective decision-making processes, such as voting, leading to a high democratic backsliding.
What if deepfakes entered the courtroom? Any visual evidence against a person would be considerably weakened, plunging the legal system into paradigms of reasoning that have not yet been explored. In such a world, Deepfakes would lead to uncertainty and constant confusion in areas that use digital content as a source of information.
This technology, initially designed for filmmaking purposes like creating a digital twin of a deceased actor to keep them in the picture, would have a much darker side.
Can this be deemed a digital pandemic?
Most deepfake programs today are open-source open source, thereby increasing the risk that this technology could become widespread. To understand the spread of deepfakes, three key events in their development may be noted:
Deepfake act 1, discovery, 2017: An anonymous Reddit user applies this technology to porn films, replacing the original faces with those of celebrities without their consent.
Deepfake act 2, raising awareness, 2018: An entirely synthetic Obama speech was generated by Jordan Peele. The generated video was so realistic that the fabrication was undetectable to the human eye. The video was meant to warn the general public about the dangers that had seeped into digital content.
Deepfake act 3, spread, 2019: A new anonymous user launched an application called “deepNude” that followed the same logic as facial manipulation, but this time for the whole body. This way, it was possible to take a dressed image of a person and make a totally naked equivalent. This application spread so quickly that the creator was forced to shut it down, and Github decided to lock access to its source code.
These three acts show the rise of multimedia content contamination. At the same time, the rendering quality has continually improved and deepfake logic has been applied to other media like voice. This environment leads to an important spread with a growing difficulty in detection.
Is there a cure for this new digital disease?
Deepfakes are trained under adversarial learning philosophy, meaning that a targeted detection program plays the role of an adversarial player to improve the quality of generation. In theory, the generator and the detector (or deepfake and anti-deepfake) called “Nash equilibrium”, where neither player has an advantage over the other.
To date, several detectors have been developed with reassuring results, but which unfortunately fall within the adversarial circle, thereby enabling a new, more advanced deepfake generation.
At present, no detector has escaped this adversarial circle.
To converge on a long-term remedy, promising initiatives have been launched in order to encourage the research community to redouble its efforts to fight deepfakes, much like the detection challenge launched by Facebook. Other challenges have since been launched, representing a sign of digital media decontamination. More recently, through its Defending Democracy Program, Microsoft announced the release of new tools to fight false content and other disinformation campaigns.
Besides the development of a remedy, collective awareness also needs to change. We must train ourselves to become informed cybercitizens. Everyone should stop and think about the digital information presented to them. Adopting a skeptical stance must become a reflex.
Any demo?
The goal here is to illustrate the key steps of generating a deepFake. I started with an existing video, one of myself, to generate deepFakes based on the faces of Emmanuel Macron, Angela Merkel, and Donald Trump.
You can see in the second video that the process of generating a deepFake uses two main steps.
The first one is training, as seen from 00:07 to 00:12s. Using a generative deep learning algorithm, the algorithm automatically learns how to encode and decode my facial characteristics compared to the face of Emmanuel Macron (red lines). This step takes a lot of computing time, possibly hours or even days to converge on an optimal state.
The second step is inference, which can be seen from 00:13 to 00:18s. This consists of using the already-trained algorithm in its optimal state to transcribe and superimpose the source faces onto my own face (green lines).
The generations made with the faces of Merkel and Trump follow the same strategy and the same color codes to help understand the steps.