Technologies Immersives & Médicales
La réalité augmentée bouleverse de nombreux secteurs industriels en offrant une nouvelle perception de l’environnement.
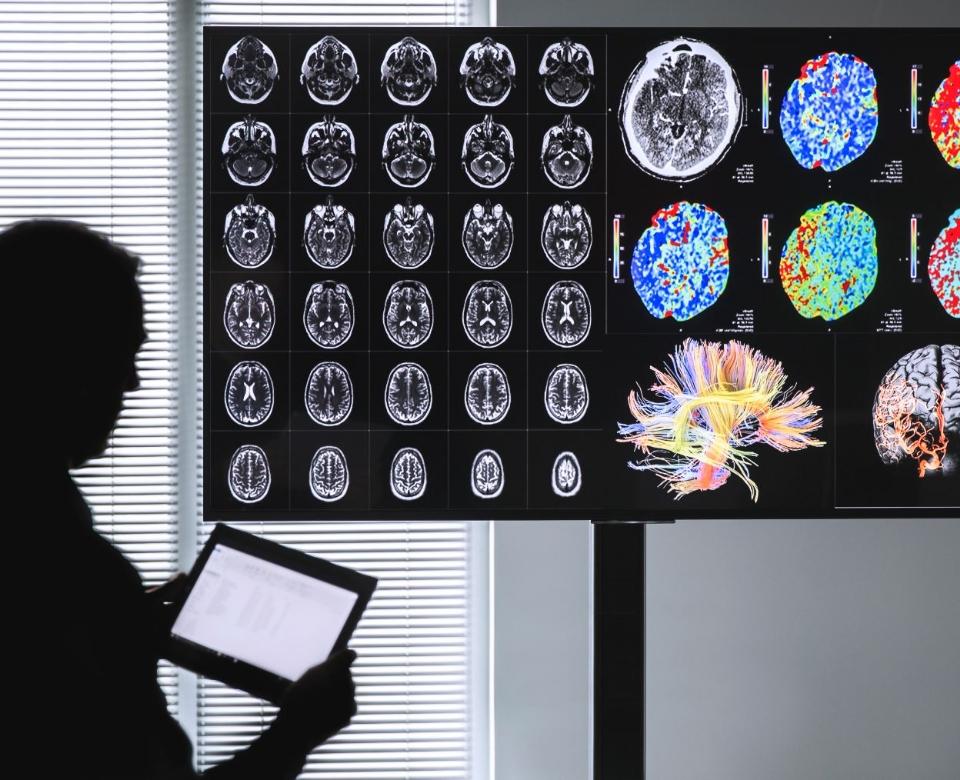
Par son expertise en vision par ordinateur, estimation de pose et visualisation 3D, le laboratoire Technologies Immersives & Médicales conçoit composants et plateformes pour augmenter les expériences des professionnels et renforcer leur efficacité, notamment grâce à la réalité augmentée et la réalité virtuelle, dans des domaines tels que l’industrie et la santé. L’utilisateur est au cœur de sa réflexion et de ses travaux, depuis la définition des besoins jusqu’à la confrontation à l’usage des technologies développées. Dans le domaine de la santé, le laboratoire travaille, avec ses partenaires médicaux, sur des technologies clefs telles que le traitement des images et des vidéos médicales, la connectivité ou l’interopérabilité.